Model | Relative share of trivial global optima | Number of trivial global optima | Number of global optima |
---|---|---|---|
QuadraticGlobalRE | 0.009 | 6625 | 714584 |
LinearGlobalRE | 0.081 | 56635 | 700830 |
QuadraticLocalRE | 0.009 | 6625 | 709289 |
LinearLocalRE | 0.07 | 50256 | 721096 |
Appendix B — Trivial Endpoints
B.1 Background
A “trivial” endpoint is a fixed point or a global optimum that consists of a singleton theory (e.g. \(T=\lbrace s_{1}\rbrace\)) and a singleton commitment (e.g. \(C = \lbrace s_{1}\rbrace\)).
Such outcomes are not bad per se, but they may be indicative of the model exploiting shortcomings in the underlying measures. In particular, “trivial” endpoints may be a consequence of the original model’s shortcoming concerning the measure of systematicity, which does not discriminate between singleton theories on the basis of the scope of theories. Note that the same shortcoming also applies to the model variants explored in this report.
B.2 Results
The results of this chapter can be reproduced with the Jupyter notebook located here.
B.2.1 Overall Results
Model | Relative share of trivial fixed points | Number of trivial fixed points | Number of fixed points |
---|---|---|---|
QuadraticGlobalRE | 0.008 | 3698 | 458147 |
LinearGlobalRE | 0.08 | 25111 | 312783 |
QuadraticLocalRE | 0.009 | 5189 | 588236 |
LinearLocalRE | 0.063 | 14443 | 228122 |
Model | Relative share of trivial fixed points | Number of trivial fixed points | Number of fixed points |
---|---|---|---|
QuadraticGlobalRE | 0.007 | 3700 | 528616 |
LinearGlobalRE | 0.08 | 25111 | 313002 |
QuadraticLocalRE | 0.006 | 11652 | 1991852 |
LinearLocalRE | 0.323 | 421058 | 1303077 |
Observations
- Overall, the relative share of trivial gobal optima (Table B.1) and fixed points (result perspective Table B.2) is very low for quadratic model variants
- Linear model variants exhibit substantially more trivial global optima, but the relative shares are still low.
LinearLocalRE
exhibits a substantial share of trivial fixed points in the process perspective (Table B.3), but not for the result perspectve (Table B.2). This indicates that relatively many branches lead to trivial fixed points.
B.2.2 Results Grouped by Sentence Pool Size
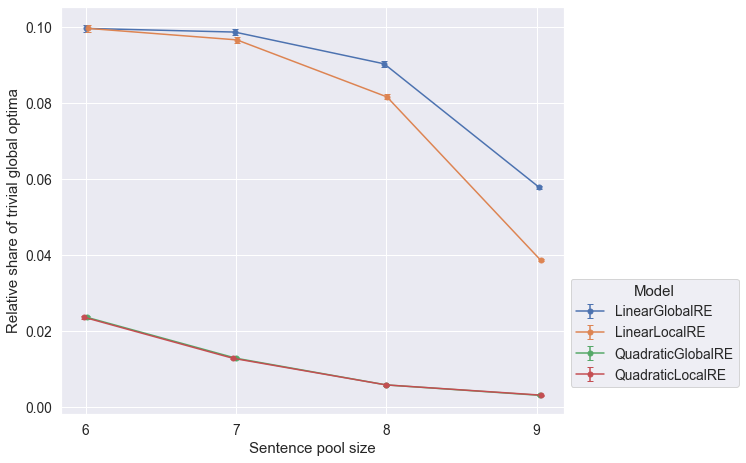
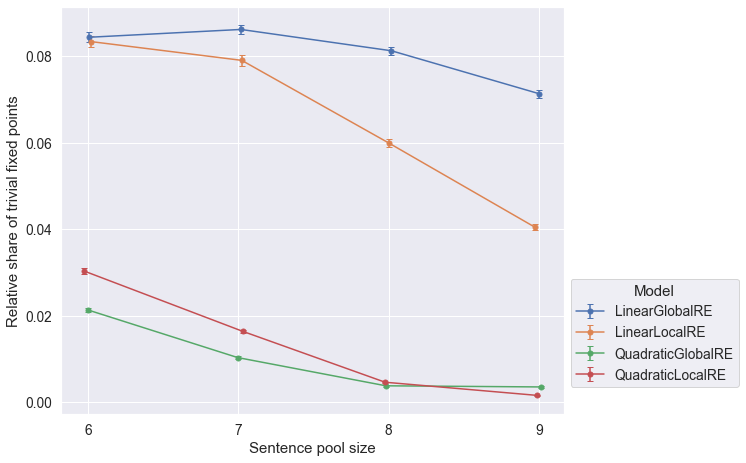
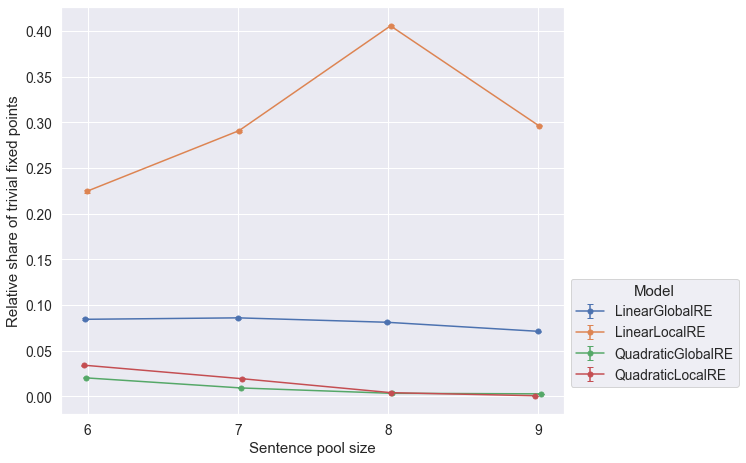
Observations
- The relative shares of trivial global optima or fixed points tend to decrease with increasing sentence pool sizes.
- A notable exception to this trend is
LinearLocalRE
in the process perspective (Figure B.3)
B.2.3 Results Grouped by Configuration of Weights
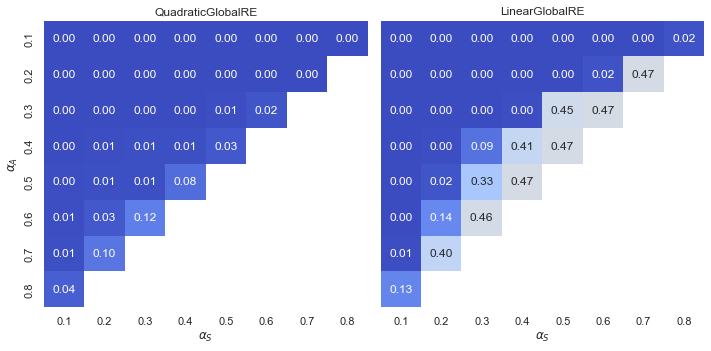
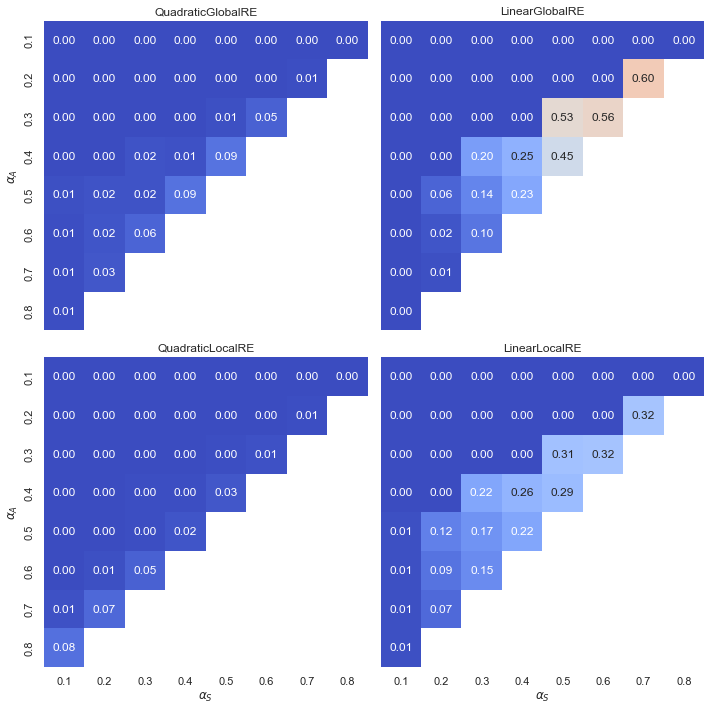
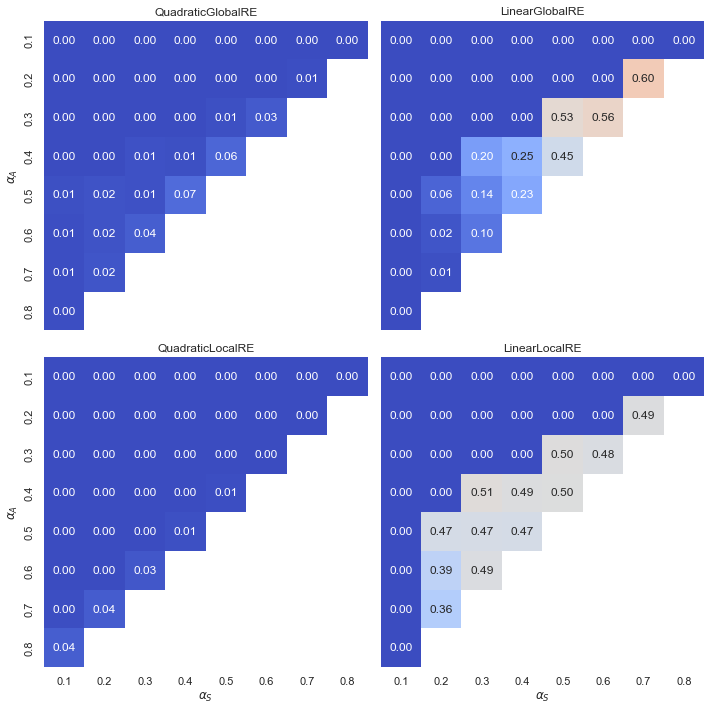
Observations
- In quadratic model variants, the configuration of weights have a small impact on the relative shares of trivial endpoints.
- Linear model variants tend to produce higher relative shares of trivial endpoints for low values of \(\alpha_{F}\) and high values of \(\alpha_{S}\).